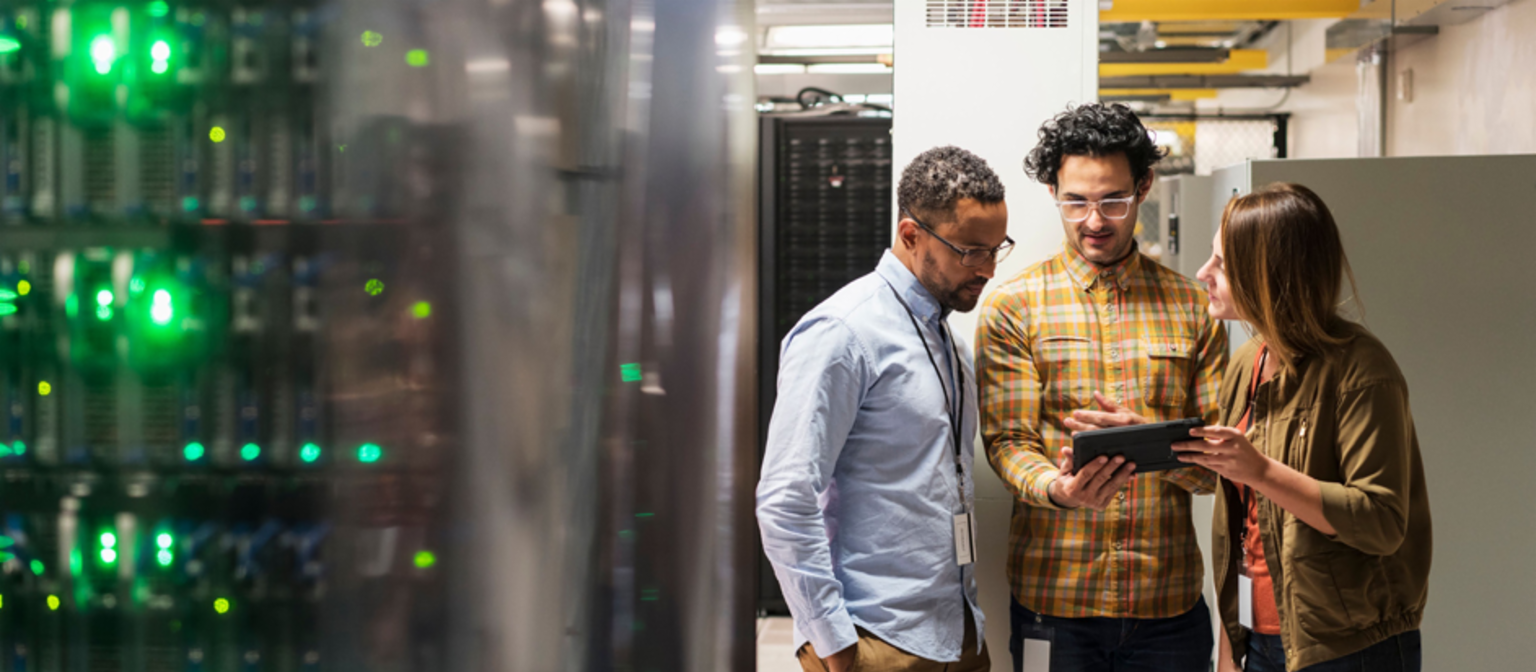
Data usage at a new level: why the financial industry needs to engage with prescriptive analytics and digital twins.
Calculating forecasts based on averages are so last year: these days, companies can perform complex live simulations for every one of their clients – using live data.
Deriving insights for the business from large volumes of data is nothing new in the financial sector. Model calculations and simulations have also been around even longer than the overused term “big data”. What is new, however, is a development that is expressed with the terms “prescriptive analytics” and “digital twins” – and it has the potential to change entire industries. Data analysis and decision-making are converging to an extent that would not have been possible before.
This is most evident with “digital twins”, a concept that comes from the manufacturing industry. In that sector, a digital twin is the computer simulation of either a single component or an entire machine. This is nothing new – engineers have long been designing components on the computer and using material properties to calculate whether the components will do what is required of them. This design data is then converted into any number of physical versions of the components.
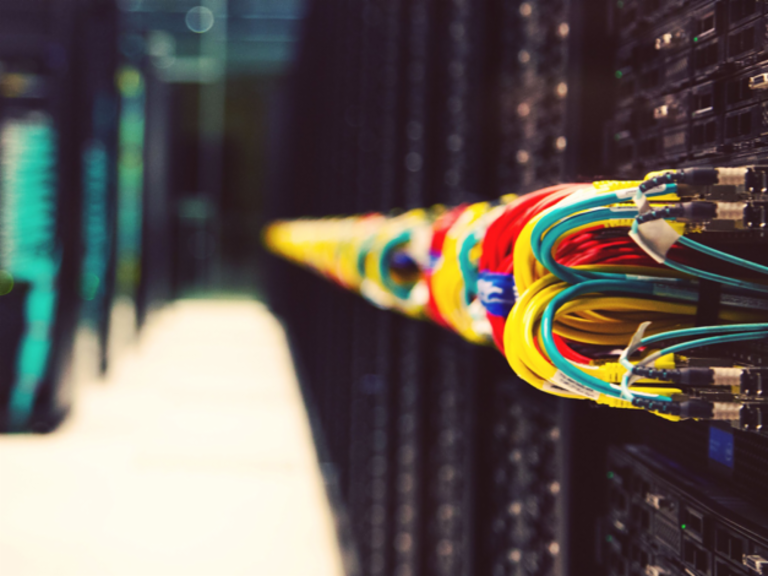
Each physical version gets its own live simulation
The data for this real-time monitoring is provided by the networked sensors in machines and components, which are becoming more and more widespread in industry – the much-cited Internet of things. According to a study by the market research firm Gartner, half of the large industrial companies will be using twin technology by 2021.
Even customers can be depicted as digital twins
Using data to predict the consequences of your own actions
The parcel service UPS, for example, uses sensors on its trucks to track their whereabouts and traffic conditions. With these findings, they optimize the routes of the delivery vehicles. Juan Perez, Chief Information Officer at UPS, explains: “If we reduce the distance for each driver in the US alone by one mile per day, we will within one year save $ 50 million per year.”